Estimation of COVID-19 cases in South American countries using ARIMA models
Keywords:
Covid-19; pandemia; predicción; modelos ARIMAAbstract
The main objective of this work is to use ARIMA models for the estimation of new contagions using public data available for Venezuela and the South American region, currently the main focus of a second COVID19 outbreak. A 30-day prediction is made for the number of Covid-19 cases in South American countries using available public data. ARIMA models were used to estimate the impact of new contagions on infection dynamics for South America Since the appearance of the first case of the new Covid-19 pneumonia in China, which has become a global public health problem and the great challenge that the infection has represented for the countries of South America to June 24, 2020, a total of 1,866,090 cases have been detected and in the particular case of Venezuela a total of 4,365 cases have been detected for the same date. The rapid increase in the number of cases and the high rate of contagion associated with the virus have led to the development of different mathematical approaches, such as: SIR, SEIR models, neural networks and linear regressions that allow predicting the probable evolution of the epidemic. The ARIMA model has been successfully used in other infections such as influenza, malaria, SARS, among others. In the following work, the 30-day prediction of the number of Covid-19 cases in South American countries is made using public data available. The results of the estimates made using these models show that even in the region, greater efforts are needed to control the epidemic.
Downloads
References
Al-Najjar, H., & Al-Rousan, N. (2020). A classifier prediction model to predict the status of Coronavirus COVID-19 patients in South Korea. European Review for Medical and Pharmacological Sciences, 24(6), 3400–3403. Recuperado en: https://doi.org/10.26355/eurrev_202003_20709
Anastassopoulou, C., Russo, L., Tsakris, A., & Siettos, C. (2020). Data-based analysis, modelling and forecasting of the COVID-19 outbreak. PLoS ONE, 15(3), 1–21. Recuperado en : https://doi.org/10.1371/journal.pone.0230405
Asteriou, D., & Stephen G., H. (2011). Applied Econometrics. Palgrave Macmillan (Second Edi). Hampshire: Palgrave Macmillan.
Bekiros, S., Kouloumpou, D., & Ceylan, Z. (2020). Estimation of COVID-19 prevalence in Italy, Spain, and France. Chaos, Solitons and Fractals, 729, 138817. Recuperado en: https://doi.org/10.1016/j.chaos.2020.109828
Box, G. E. P., Jenkins, G. M., & Reinsel, G. C. (2013). Time series analysis: Forecasting and control: Fourth edition. Wiley Series in Probability and Statistics (Vol. 37). Wiley. Recuperado en : https://doi.org/10.1002/9781118619193
CHOI, K., & THACKER, S. B. (1981). An Evaluation of Influenza Mortality Surveillance, 1962–1979. American Journal of Epidemiology, 113(3), 215–226. Recuperado en: https://doi.org/10.1093/oxfordjournals.aje.a113090
Du, R. H., Liang, L. R., Yang, C. Q., Wang, W., Cao, T. Z., Li, M.,Shi, H. Z. (2020). Predictors of mortality for patients with COVID-19 pneumonia caused by SARSCoV- 2: A prospective cohort study. European Respiratory Journal, 55(5), 2000524. Recuperado en: https://doi.org/10.1183/13993003.00524-2020
Ghosal, S., Sengupta, S., Majumder, M., & Sinha, B. (2020). Prediction of the number of deaths in India due to SARS-CoV-2 at 5–6 weeks. Diabetes and Metabolic Syndrome: Clinical Research and Reviews, 14(4), 311–315. Recuperado en: https://doi.org/10.1016/j.dsx.2020.03.017
Giordano, G., Blanchini, F., Bruno, R., Colaneri, P., Di Filippo, A., Di Matteo, A., & Colaneri, M. (2020). Modelling the COVID-19 epidemic and implementation of population-wide interventions in Italy. Nature Medicine, 26(June). Recuperado en: https://doi.org/10.1038/s41591-020-0883-7
Guidotti, E., & Ardia, D. (2020). COVID-19 Data Hub. Recuperado en: https://doi.org/10.13140/RG.2.2.11649.81763
Hyndman, R., & Khandakar, Y. (2008). Automatic Time Series Forecasting: The forecast Package for R. Journal of Statistical Software, Articles, 27(3), 1–22. Recuperado en: https://doi.org/10.18637/jss.v027.i03
Khan, A. I., Shah, J. L., & Bhat, M. M. (2020). CoroNet: A deep neural network for detection and diagnosis of COVID-19 from chest x-ray images. Computer Methods and Programs in Biomedicine, 196, 105581.Recuperado en: https://doi.org/10.1016/j.cmpb.2020.105581
Lauer, S. A., Grantz, K. H., Bi, Q., Jones, F. K., Zheng, Q., Meredith, H. R., … Lessler, J. (2020). The Incubation Period of Coronavirus Disease 2019 (COVID-19) From Publicly Reported Confirmed Cases: Estimation and Application. Annals of Internal Medicine, 172(9), 577–582. Recuperado en: https://doi.org/10.7326/M20-0504
R Core Team. (2020). R: A Language and Environment for Statistical Computing. Vienna, Austria. Recuperado en: https://www.r-project.org/
RStudio Team. (2020). RStudio: Integrated Development Environment for R. Boston, MA. Recuperado en: http://www.rstudio.com/
Said, S. E., & Dickey, D. A. (1984). Testing for Unit Roots in Autoregressive-Moving Average Models of Unknown Order. Biometrika, 71(3), 599–607. Recuperado en: https://doi.org/10.2307/2336570
Trapletti, A., & Hornik, K. (2019). tseries: Time Series Analysis and Computational Finance. Recuperado en: https://cran.r-project.org/package=tseries
Wang, C., Horby, P. W., Hayden, F. G., & Gao, G. F. (2020). A novel coronavirus outbreak of global health concern. The Lancet. Recuperado en: https://doi.org/10.1016/S0140-6736(20)30185-9
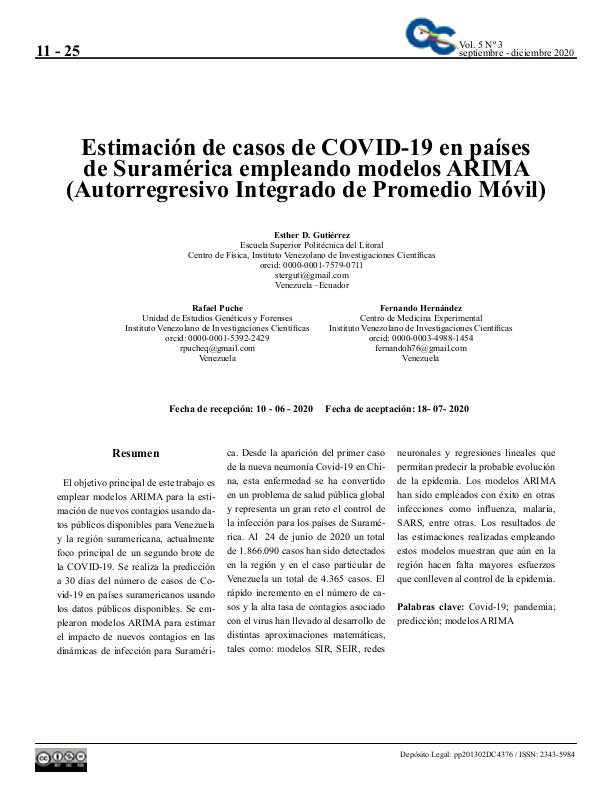
Downloads
Published
How to Cite
Issue
Section
License

This work is licensed under a Creative Commons Attribution-NoDerivatives 4.0 International License.